Articles
Google Pmax Scripts
Optimizing Google Pmax campaigns can be a headache 🤯 , especially when it comes to understanding where the spending is allocated. Leverage Propel Digital's Pmax script to efficiently allocate costs across various placements such as search, video, and shopping.
https://propeldigital.com/free-scripts/performance-max-reporting-script/
Learn how to run AdWords scripts effectively with this guide:
https://developers.google.com/google-ads/scripts/docs/getting-started
Once you've mastered cost allocation, explore standalone campaigns in search, video, and display to gauge your Return on Ad Spend (ROAS) and Customer Acquisition Cost (CAC) metrics.
Happy scripting and optimising your campaigns!
Credits:Propeldigital
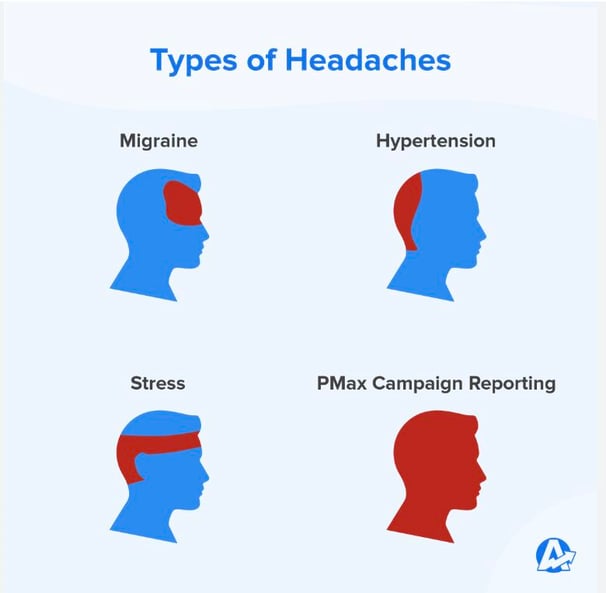
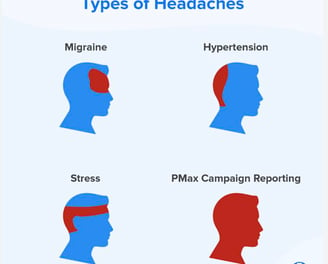
Incrementality Measurement: The Next Big Thing in Marketing
In the ever-evolving world of digital marketing, a new star is rising on the horizon: incrementality measurement. As marketers, we're constantly seeking ways to prove the value of our efforts and optimize our strategies. Incrementality measurement promises to do just that, offering a more accurate and nuanced understanding of marketing impact than ever before.
What is Incrementality Measurement?
At its core, incrementality measurement answers a crucial question: "What additional value did this marketing activity generate that wouldn't have happened otherwise?" Unlike traditional attribution models that focus on touchpoints along the customer journey, incrementality measurement isolates the true impact of marketing efforts by comparing the behavior of exposed groups to control groups.
Why It's a Game-Changer
True ROI Insights: By measuring the incremental impact of marketing activities, businesses can finally understand the real return on their marketing investments. This goes beyond simple correlation to establish causation.
Eliminating Waste: Incrementality measurement helps identify which marketing efforts are truly driving new business versus those that are reaching customers who would have converted anyway. This insight allows for more efficient budget allocation.
Holistic View: Unlike channel-specific metrics, incrementality provides a cross-channel view of marketing effectiveness, accounting for both online and offline touchpoints.
Overcoming Attribution Challenges: In a world where customer journeys are increasingly complex and privacy regulations are tightening, traditional attribution models are falling short. Incrementality measurement offers a privacy-friendly alternative that doesn't rely on individual-level tracking.
Why Now?
Several factors are converging to make incrementality measurement more relevant and accessible than ever:
Advanced Analytics: Improvements in data processing and machine learning have made it possible to conduct incrementality tests at scale.
Economic Pressures: In times of economic uncertainty, businesses are under increased pressure to justify marketing spend, making incrementality insights particularly valuable.
Digital Saturation: As digital channels become more crowded, understanding the true impact of each marketing effort becomes crucial for standing out.
Challenges and Considerations
While incrementality measurement offers exciting possibilities, it's not without challenges. Implementing it requires a shift in mindset, potentially significant changes to measurement infrastructure, and a willingness to experiment. It also demands careful interpretation of results and the ability to act on insights.
The Future of Marketing Measurement
As we look to the future, incrementality measurement is poised to become an essential tool in the modern marketer's toolkit. It promises to bring greater accountability to marketing spend, drive more informed decision-making, and ultimately lead to more effective marketing strategies.
For marketers who want to stay ahead of the curve, now is the time to start exploring incrementality measurement. By embracing this approach, you'll be better equipped to prove and improve the value of your marketing efforts in an increasingly complex and competitive landscape.
In conclusion, incrementality measurement isn't just a passing trend – it's the next big thing in marketing, offering a more accurate, nuanced, and actionable understanding of marketing impact. As the marketing world continues to evolve, those who master incrementality measurement will find themselves with a significant competitive advantage.
Creative Scoring: A Fixed Methodology for Evaluating Marketing Ads
Why Creative Scoring Matters
Marketers often measure the success of ad creatives using KPIs like CPM (Cost Per Mille), CTR (Click-Through Rate), and CPI (Cost Per Install). However, manually evaluating creatives can be inconsistent. With a fixed methodology, we establish thresholds for these KPIs and apply a classification system to determine whether a creative is performing well or not.
Data Preparation
To begin, we need a structured dataset containing ad performance metrics. Our dataset includes the following key columns:
Amount Spent (EUR): The total ad spend
CPM: Cost per 1,000 impressions
CTR: Click-through rate
CPI: Cost per install
Data Cleaning
Before applying our classification model, we clean and preprocess the data:
Remove unnecessary columns such as preview links and reporting dates.
Standardize column names to lowercase and replace spaces with underscores.
Handle missing values by replacing them with zeros.
Convert numeric columns to float format for consistent calculations.
Defining KPI Thresholds
A fixed methodology requires pre-determined benchmarks for classification. We set the following KPI thresholds:
CPM: Should be ≤ €9.00 (Lower is better)
CTR: Should be ≥ 0.50% (Higher is better)
CPI: Should be ≤ €3.00 (Lower is better)
Implementing the Classification Model
To categorize creatives as 'Good' or 'Bad', we define a scoring function:
Compare each metric with the threshold.
Assign a score (1 for meeting the threshold, 0 otherwise).
Compute an overall score as the average of individual KPI scores.
Classify creatives:
Good: If the overall score is ≥ 0.6
Bad: If the overall score is < 0.6
Additionally, we apply color coding for visualization:
Green for good-performing creatives.
Red for underperforming creatives.
Results and Insights
After applying our classification function to the dataset, we gain actionable insights:
Percentage of ads meeting each KPI: This helps identify which metric needs optimization.
Overall creative performance distribution: Marketers can focus on improving underperforming ads.
Data-driven decision making: Instead of relying on intuition, ad creatives are evaluated consistently.
Conclusion
By using a fixed creative scoring methodology, marketers can systematically evaluate ad performance. This approach not only streamlines the optimization process but also ensures that budget allocation is data-driven and impactful.
Here is the link to github: https://github.com/rakeshtparayil/Machine_Learning_Marketing/blob/main/Creative_Scoring_Fixed_Methodolgy.ipynb